TABLE OF CONTENTS
Impact of generative AI on DE&I
While generative artificial intelligence (AI) has existed since the 1960s, it has recently gained significant attention. Notably, there have been reports that an organisation’s recruitment process is now entirely AI-driven. This whitepaper captures insights from a roundtable discussion held at the Hays Birmingham Office in June 2024, where participants explored the impact of AI on diversity, equity, and inclusion (DE&I). Participants also discussed strategies to mitigate risks and biases, emphasising the importance of promoting inclusion for all.
Robotic Process Automation (RPA) vs AI vs GenAI
It is important to establish the key differences between RPA, AI and generative AI.
Robotic Process Automation (RPA) is about automating repetitive and mundane tasks across business applications and systems within an organisation. Traditionally, RPA relies on predefined rules and sets of workflows consisting of repetitive rule-based instructions that humans execute on machines.
Artificial Intelligence (AI) refers to the development of computer systems that can perform tasks typically requiring human intelligence. These systems learn from data, adapt to new information, and make decisions based on patterns and algorithms. AI encompasses various subfields, including machine learning, natural language processing, and computer vision.
Generative AI (GenAI) is dedicated to producing new content, such as communications, images, or even music, using patterns and data that it has been trained on. Generative AI models can produce new information that is synthesised in a way that resembles human creativity rather than simply copying the given data.
- RPA works best when it’s used to handle rule-based processes where the workflows don’t change over time.
- AI can easily handle complex processes that previously could only be carried out by humans alone. This is because AI robots can make cognitive decisions using large data sets to predict several possible outcomes.
- Generative AI is a shift from enabler of our work to potential co-pilot
What are the risks AI poses to DE&I?
Biased training data
Generative AI outputs can inadvertently perpetuate biases present in their training data, algorithms, and other guiding inputs. Tools such as Midjourney and ChatGPT were trained on extensive online data, which unfortunately included some potentially harmful and biased content that may be included in output.
Additionally, company data can reflect historically biased decisions. Individuals from specific groups may have faced lower hiring rates or previously received less positive feedback. AI models lacking diverse, high-quality training data and mechanisms to identify concerns may inadvertently reproduce these biased patterns from company
records.
- Recruiting and hiring: Amazon’s experimental AI recruiting tool downgraded female candidates’ resumes because it was trained on predominantly male profiles.
The digital divide
There exists an opportunity gap between those who can access new technologies and those who cannot. The internet, mobile devices, and AI unlock new resources and capabilities, but those who lack access won’t benefit. AI necessitates a computer or mobile device, which many people either cannot afford or will not receive from their employers unless it’s essential for their work. The digital divide also relates to exposure. Effective use, understanding, and benefit from AI require hands-on learning. Individuals who don’t have access to AI at home, in school, or in their jobs won’t experience the same professional growth as those who do.
Data privacy and security
Without proper controls, integrating AI with company systems poses risks to employee data privacy and security. Colleagues might inadvertently access each other’s private information and AI vendors could mishandle personal data from customers and employees, leaving it vulnerable to cyberattacks and identity theft by third parties.
Lack of diversity in AI development teams
The impact of a lack of diversity in AI development teams is far-reaching. Without diverse perspectives, AI algorithms and data can reflect the biases and assumptions of their creators, perpetuating existing inequalities and potentially harming marginalised groups. Additionally, flaws in the working culture of AI can lead to biased technologies that exclude and harm entire populations, resulting in flawed ‘intelligence’ lacking varied social-emotional and cultural knowledge. Addressing diversity and inclusion in AI development is crucial for building fair, trustworthy, and effective systems.
What opportunities does AI bring to DE&I?
Boosted efficiency
It’s no secret that DEI programs are under strain, and leaders in this field are feeling burned out, exhausted from shouldering the entire load. AI can alleviate the burden on DEI leaders and optimise costs. By automating tasks such as drafting content and reporting on DEI metrics, AI streamlines processes, freeing up time for Heads of DEI and their teams to achieve more with existing resources. And it’s not just those in the DEI function who stand to benefit.
Improved engagement
AI plays a pivotal role in fostering DE&I through enhanced engagement. Recruiters can leverage AI to attract and engage diverse talent by implementing inclusive strategies, refining outreach efforts, and creating more welcoming job descriptions and interview processes. Generative AI further contributes by monitoring employee sentiment and performance, guiding management to better support and retain talent from underrepresented groups. This coaching may involve training simulations for
interacting with different segments of the workforce. Additionally, AI enhances the overall employee experience through personalised engagement.
Applying a DE&I lens
Analysing company data for bias and inequity across every employee and dimension of diversity may seem like a daunting task. However, AI has transformed this process. It can efficiently process massive datasets in a fraction of the time it would take most individuals. Similarly, auditing communications through a DE&I lens is critical but challenging. Corporate messages are often planned well in advance, yet inclusive language and cultural contexts evolve dynamically. AI enables organisations to adapt, identify concerns, and fine-tune their messaging for maximum impact.
Reducing barriers
Generative AI holds immense promise when it comes to breaking down barriers. By analysing data and creating information on users’ behalf, it bridges knowledge and skills gaps. For instance, it enhances navigation, streamlines drafting processes, aids memory retention, and provides concise summaries—all of which benefit individuals who identify as neurodivergent. Importantly, these advancements aren’t exclusive; they positively impact everyone. Consider tools such as Word, which assess the readability of documents using AI. Chances are, you’re already benefiting from AI designed to make information more accessible. This levelling of the playing field opens up new career opportunities for a wider range of people, especially those from underserved populations.
AI tools that help to promote DE&I
AI tools play a pivotal role in promoting DE&I by automating tasks, analysing data, and ensuring fair decision-making. Let’s explore three key areas where AI contributes:
- Bias-free recruitment:
- AI mimics blind recruitment processes but with added rigor. It adheres strictly to preferred metrics, reducing human bias.
- By relying on objective criteria, AI helps level the playing field for all applicants.
- Bias-free promotion/selection:
- AI assesses employees’ skills and readiness for higher positions without preconceptions. It removes bias and expectations. Breaking the glass ceiling becomes more feasible with this unbiased approach.
- Diverse interview panels:
- AI algorithms randomly assemble interview panels and pair applicants with committee members.
- This double-blind system mitigates interviewer biases, ensuring fair evaluations.
- While AI minimises bias, it’s essential to acknowledge that developers themselves may have biases. However, these personal prejudices do not necessarily permeate the AI system.
Machine learning filtering top applicants
In modern talent acquisition, data-driven recruitment software leverages machine learning to identify high-potential applicants for specific roles. By assessing skills and essential traits, this system ensures an objective match between candidates and job requirements. Importantly, it eliminates identifiers such as race, ethnicity, sexual orientation, and age, which can introduce bias.
Furthermore, automating repetitive processes and analytics liberates HR teams. With machines handling routine tasks, people teams can concentrate on strategic initiatives that enhance the overall employee experience.
AI-based pay grade evaluation
Corporate bias becomes evident in the disparities among employees’ pay. This blatant discrimination is particularly pronounced when individuals are denied opportunities for career advancement. To address this issue, organizations can implement an AI-based system to evaluate employee salaries.
Key features of the AI-based salary evaluation system:
- Objective assessment: The system assesses an employee’s skills, performance, and responsibilities without subjective biases.
- Fair compensation: Employees receive a salary range that genuinely reflects their contributions to the company.
Transparency:
The system considers only mandated deductions and benefits, ensuring transparency and equity. By leveraging AI, companies can create a more just compensation structure, benefiting all employees.
Ethical considerations in AI
Ethical considerations in AI encompass a wide range of topics, including algorithmic biases, fairness, automated decision-making, accountability, privacy, and regulation. These considerations are crucial for ensuring responsible and equitable use of artificial intelligence. Codes of ethics in companies and government-led regulatory frameworks are two main ways that AI ethics can be implemented.
Accountability: who is responsible for AI decisions?
Businesses integrating AI into their operations must establish well-defined guidelines for its use. They bear responsibility for the outcomes of AI implementation within their organisation, necessitating robust risk management strategies and incident response plans. Managers play a crucial role in ensuring their teams receive proper training to use AI responsibly. Additionally, they are accountable for monitoring AI usage, ensuring alignment with the company’s AI policy and guidelines.
Other areas for consideration include:
- Legal framework and compliance: ensure compliance with relevant data protection laws and regulations.
- Ownership and accountability: clearly define who owns the policy related to AI decisions.
- Organisational policies and procedures: develop policies that specify rules for explaining AI-assisted decisions. These policies should address what, why, and who
is responsible for providing explanations to affected individuals. - Roles and documentation: assign roles within your organisation to handle meaningful explanations.
- Transparency and fairness: prioritise transparency and fairness in AI decisions.
Transparency: how to ensure AI decision-making is fair
Ensuring fair AI decision-making involves clear rules, accountability, transparency, bias mitigation, and ethical impact assessment. Outlined below are some key steps to
ensure decision making is fair for all.
- Transparency: make the decision-making processes of AI systems clear and understandable
- Accountability: hold developers and users responsible for the outcomes of AI applications
- Ethical consideration: prioritise ethical guidelines to prevent biases and discriminatory outcomes
- Diverse training data: ensure training data represents diverse populations to avoid bias
- Regular audits: conduct regular audits of AI systems to detect and correct biases.
Fairness: how to guarantee AI benefits all groups equally
Ensuring that AI benefits all groups equally is a multifaceted challenge that requires a comprehensive approach. Here are some key strategies:
- Diversity in AI development: it’s crucial to have diverse teams involved in the ideation, design, and development of AI systems. This helps to ensure a variety of perspectives and experiences are considered, reducing the risk of bias.
- Inclusive data sets: AI systems should be trained on data sets that represent a wide range of demographics. This helps to ensure that the AI’s predictions and decisions are applicable and fair to all groups.
- Transparent and accountable AI governance: establishing clear frameworks for AI governance can help ensure that AI systems are used ethically and responsibly. This includes principles and guidelines for fairness, transparency, and accountability.
- Regular impact assessments: conducting regular assessments of AI systems can help identify and mitigate biases. This includes reviewing AI initiatives continuously for unintended outcomes and potential transformational business improvements.
- Education and awareness: organisations should educate their people on AI and how to be ready for the opportunities and challenges it presents. This could be done through setting up a body that is a visible focus for AI, such as a centre of excellence.
- Engagement with diverse stakeholders: engaging with diverse stakeholders and communities throughout the AI system’s lifecycle can help ensure that the technology addresses a broad spectrum of needs and challenges.
By implementing these strategies, we can work towards ensuring that AI systems are developed and used in a way that benefits all groups equally. However, it’s important to remember that this is an ongoing process that requires continuous effort and vigilance.
The role of business in AI and DE&I
AI will transform the landscape of work, doing so in a manner that elevates DE&I to a fundamental expectation and a vital necessity for companies aiming for sustained growth. Consequently, businesses should prioritise the following strategies when designing and implementing AI systems:
Develop ethical AI guidelines
You need clear ethical guidelines and policies for your colleagues to follow when they use artificial intelligence in their day-to-day work. Rulebooks mean structure, and structure is crucial to success. Not only do you need to establish these – you also must enforce them, with clear information on potential risks, ethical considerations and especially compliance requirements to ensure that AI is implemented responsibly.
Invest in DE&I training and initiatives
Provide training, mentorship and career advancement opportunities to underrepresented employees, helping them grow professionally and contribute to AI development.
Collaborate with diverse communities
To create a well-designed AI system, it’s essential to involve a wide range of stakeholders, from different demographic groups and backgrounds. This will help establish a system that is fair and transparent, respects diversity and different cultures, and can be easily accessed by all user groups. Also, training the AI system on data that reflects the complexity, diversity and cultural richness of the real world is critical.
Learning themes and top tips
Participants at our event were divided into groups and asked to answer the following questions.
- Transparency and accountability: what can we do to make AI decision-making processes transparent and accountable?
- Fairness metrics: what metrics can we use to assess equitable outcomes for all?
- Ethical guidelines: what are the key steps to establishing clear ethical guidelines for AI deployment, regarding areas like hiring?
- Equitable access: how do we ensure that AI benefits all users, regardless of their background or abilities?
Themes explored
Transparency andaccountability: what can we do to make AI decision making processes transparent and accountable? |
Fairness metrics: what metrics can we use to assess equitable outcomes for all? |
Ethical guidelines: what are the key steps to establishing clearethical guidelines for AI deployment, regarding areas like hiring? |
Equitable access: how do we ensure that AI benefits all users, regardless of their background or abilities? |
Evaluate models for fairness by analysing their impact across different demographic groups | Conduct random checking of results | Develop a strategy and ensure your vision is clear and explainable | Understand what an equitable process looks like |
Use frameworks of operations. Once information is produced | Use frameworks of operations. Once information is produced – clarify who decides how it is used | Conduct comparisons to census data | Prioritise data privacy and security |
Conduct audits and checks, remembering to bring in human check points into the process | Monitor goals and measure percentage of employees in roles | Train employees on ethical AI use | Understand your users - ensure human access routes are available to capture those who may not have ready access to AI |
Outline appropriate training – is it mandatory, if so, how often is it organised? | Promote transparency by communicating progress on agreed targets and staff engagement results |
Assemble a diverse ethical AI task force | Ensure the training team is diverse and able to keep the system functioning effectively |
Ensure adherence to relevant legislation and governance regulations | Implement ethical design and development practices |
To find out how we can support your diversity and inclusion aspirations please contact us.
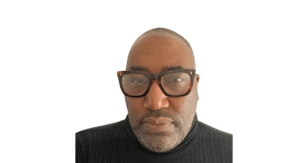